IMPACT 50 Recognition
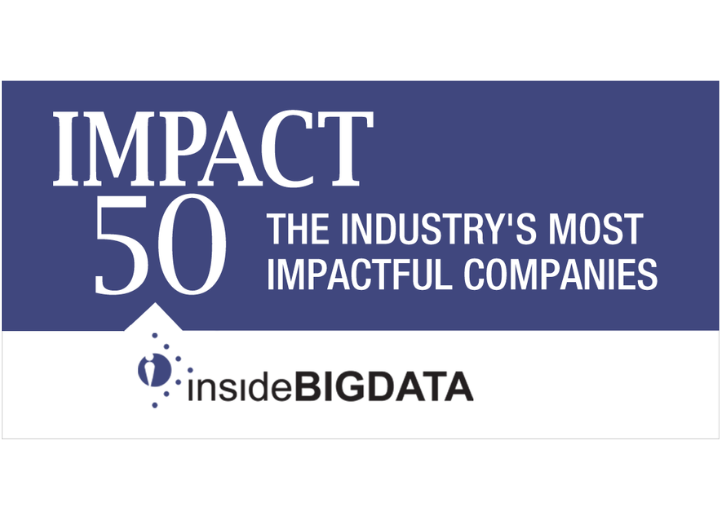
Daniel Rodriguez
In the rapidly evolving field of artificial intelligence, prompt engineering has emerged as a crucial skill for developers, data scientists, and researchers working with large language models (LLMs). As we harness the capabilities of models like GPT-4, understanding the fundamentals of prompt engineering, key techniques to use, and best practices to follow can help you unlock the full potential of LLMs.
Prompt engineering is the art and science of designing input prompts that elicit desired responses from LLMs. It involves crafting precise instructions, providing context, and structuring queries to guide the model toward generating accurate, relevant, and useful outputs. Essentially, it’s about communicating effectively with AI to achieve specific goals—whether that means answering a question, generating creative content, or extracting insights from data.
Large language models are incredibly powerful, but they are highly dependent on the prompts users provide.
A well-crafted prompt can lead to insightful, accurate responses, while a poorly designed one may result in irrelevant or misleading outputs. Prompt engineering bridges the gap between human intent and machine understanding, enabling more effective and controlled use of these models across a variety of applications—from automating workflows to generating novel content.
The impact of well-crafted prompts is clear in a study by Anthropic on contextual retrieval, where combining specific techniques reduced retrieval failure rates significantly. Similarly, IBM emphasizes that well-designed prompts help ensure AI responses are accurate and pertinent, directly improving user satisfaction.
Here are some foundational techniques and patterns that can enhance your prompt engineering skills:
This kind of prompting encourages the model to break down complex problems into step-by-step reasoning processes.
Example: Explain step-by-step how photosynthesis works.
Expected output:
This prompt clearly states the task and desired output format. This approach can significantly improve results.
Example: Summarize the following article in three bullet points.
When you use this prompt, you are assigning a specific role to the AI that can help focus its responses.
Example: You are a cybersecurity expert. Provide advice on how to protect personal data online.
This prompt provides relevant background information and enhances the model’s understanding and output quality.
Example: Based on the following data trends, predict the next quarter’s sales.
These techniques, while seemingly straightforward, can lead to remarkable improvements when applied systematically.
A study by Clavié et al. demonstrated how prompt engineering techniques significantly boosted the performance of GPT-3.5 in classifying job postings. Their experiments with zero-shot, few-shot, and chain-of-thought prompting raised accuracy from 65.6% to 91.7%. This highlights the transformative impact of strategic prompt design.
Prompt engineering is not limited to text. It extends to other modalities, expanding the capabilities of AI beyond language.
Platforms like DALL-E 3, Midjourney, or Adobe Firefly allow the generation of images with text prompts.
Example: Create an image of a futuristic city with flying cars and towering skyscrapers.
Using prompts to produce or manipulate audio and video content is an emerging field:
Crafting effective prompts is an iterative process that significantly affects the quality of output generated by large language models. Through careful evaluation and refinement, prompts can be transformed from producing generic or imprecise results to delivering nuanced and highly relevant responses. This process often involves multiple iterations, where each adjustment seeks to align the model’s responses more closely with the desired outcomes.
One descriptive approach to refining prompts involves examining several dimensions of model interaction:
Evaluating and refining prompts can lead to discovering patterns that work consistently well for certain tasks, which helps in building a repository of effective prompts. This repository then becomes a foundational tool that accelerates future development efforts, leveraging accumulated knowledge to avoid starting from scratch each time.
In our work at Anaconda, we applied an Evaluations Driven Development (EDD) methodology, powered by our in-house “llm-eval” framework, to optimize our prompt designs. EDD consists of rigorously testing and refining the prompts and queries we use to elicit relevant, reliable outputs from the underlying language models. Rather than just optimizing for abstract benchmarks, we evaluate the Anaconda AI Assistant on its ability to handle the actual challenges faced by data scientists in their daily work. Through this process, we achieved a significant increase in precision.
Several tools and frameworks can assist with prompt engineering:
These tools offer pre-built components, templates, and utilities that make the prompt engineering process more streamlined and efficient by reducing the complexity of designing, testing, and integrating prompts. They help developers focus on the core aspects of prompt effectiveness rather than spending time on repetitive, low-level implementation details.
While prompt engineering unlocks powerful capabilities, it’s essential to consider ethical implications in the use of large language models:
As large language models continue to advance, the way users interact with them will become simpler and more intuitive, leading to major improvements in usability and efficiency.
Imagine models that understand what you need without requiring detailed instructions. These adaptive models will be able to infer more context on their own, meaning you won’t have to be an expert in crafting precise prompts to get the best results.
Tools that can automatically create effective prompts for you based on your goals will soon become available, making it easier for anyone to leverage the power of AI without needing deep technical knowledge. This means that, instead of spending time figuring out the best way to ask a question, you can focus more on solving your problem.
At the same time, as the models become better at handling multiple types of inputs—text, images, audio, and even video—they will open up new possibilities for creating rich, interactive experiences. You’ll be able to engage with AI in more natural and creative ways, making complex tasks feel effortless.
Prompt engineering is a vital skill in the era of large language models. By mastering the techniques and best practices outlined in this article, you can harness the full power of LLMs to create sophisticated and capable AI applications. As the field grows, staying informed and continually refining your skills will keep you at the forefront of AI innovation.
Ready to start prompting? Experiment with the techniques discussed using your preferred LLM platform. Explore the tools mentioned to streamline your workflow, and join communities focused on prompt engineering to share insights and learn from others.
Talk to one of our experts to find solutions for your AI journey.